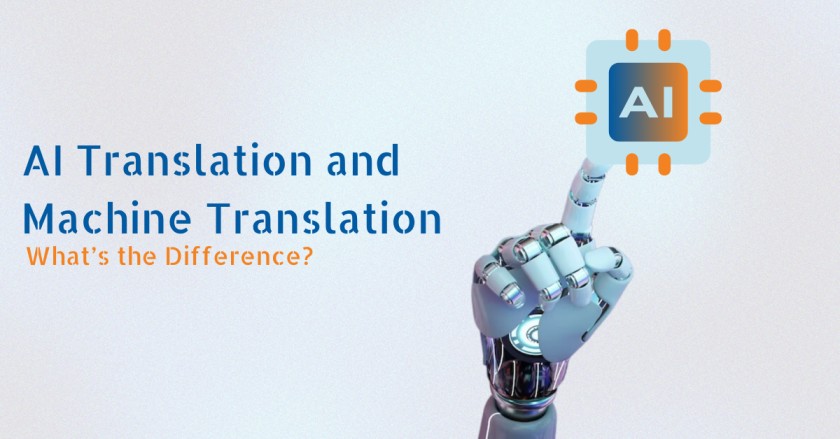
AI Translation and Machine Translation: What’s the Difference?
The worldwide translation industry is
predicted to reach $51 billion by 2031, driven by the soaring demand for
translation services. As organisations expand globally and digital content
spreads across languages, traditional human translation cannot keep up with the
volume of content that needs to be translated.
Recent research shows that although just
25.9% of online information is available in English, over 70% of users prefer
to view items in their native tongue. Two important technology
solutions—machine and artificial intelligence translations—have emerged from
this discrepancy. Although these words are used synonymously most of the time,
they reflect different methods of automated language translation.
Understanding AI Translation
Driven by sophisticated neural networks and
deep learning algorithms, artificial intelligence translation marks the front
edge of language technologies. These technologies grasp context, cultural
quirks, and industry-specific language in addition to translating words. Modern
AI translation systems use natural language processing (NLP) to figure out what
phrases and idioms mean instead of translating them word-for-word.
For example, when an AI translation system
comes across the English phrase "it's raining cats and dogs," it
recognises it as an idiom and can produce a culturally acceptable equivalent in
the target language rather than a word-for-word translation. Learning from
mistakes and feedback, artificial intelligence translating systems also
constantly increase their accuracy and grasp of linguistic nuances.
What is Machine Translation?
Using statistical or rule-based techniques
to translate text from one language to another, machine translation (MT)
reflects the more conventional approach to automated translating. It means that
these systems make their translation based on pre-operational linguistic rules
and the statistical tendencies. The most famous one may be Google Translate
which started with statistical machine translation and then adopted neural
machine translation.
Machine translation divides phrases into
segments and translates segment-bases. With the help of rules and regulations
set by the language translator, they then translate them in the target
language. This method can be used when the material is just simple and
straightforward, but it poses serious problems when applied to sentences,
idioms, or even where the same word may have a different meaning in different
contexts.
Key Differences Between AI Translation
and Machine Translation
1. Specialization
AI Translation: Advanced artificial
intelligence translation has the capability of being trained on enterprise type
content and therefore can perform better in professional areas of legal,
medical and technical translation. They understand terms of reference and
context, having an affinity with professional and technical writing.
Machine Translation: Most previous MT
systems can be termed as rigid in the sense that they apply similar translation
procedures whether or not the content is of a specific type. This can lead to
less accurate translations in some specialised areas where it is critical to
use the exact terms.
2. Methods of Training
AI Translation: Modern artificial
intelligence translating systems use neural networks learning from enormous
volumes of high-quality, human-validated translations. By using machine
learning, they keep getting better, adjusting to new language patterns and
changing use. These algorithms can grasp context and, depending on the whole
context of the content, make wise word choice judgements.
Machine Translation: Traditional MT systems
are based on pre-programmed rules and statistical patterns. They do not
"learn" in the same way artificial intelligence systems do, even
while they can be changed with fresh rules and terminology. Their development
calls for hand-written updates and regulation changes.
3. Translation Quality
AI Translation: AI-powered translation
produces more natural-sounding and context-aware translations. These systems
conserve formatting, grasp document structure, and keep consistency over
extended papers. They especially get rather good with cultural references and
idioms.
Machine Translation: MT systems often
generate more literal translations that might sound mechanical or unnatural.
They usually struggle with complicated phrase patterns and may overlook
critical contextual information, resulting in potential misunderstandings or
incorrect phrasing.
4. Application Range
AI Translation: AI translation systems
excel at handling a wide range of content categories, including imaginative
marketing materials and technical paperwork. They can tailor their translation
style to the content type and target audience, making them appropriate for both
professional and informal content.
Machine Translation: Machine translation
works best for simple, uncomplicated text that can be translated literally.
It's usually used for simple communication or getting the main idea of a text,
not for making polished translations that are ready for release.
5. Convenience and Integration
AI Translation: Modern artificial
intelligence translating systems can include sophisticated tools including
quality assurance tools, translating memory, and terminology management. For
major translation projects, they are quite effective since they can be included
in tools for workflow automation and content management systems.
Machine Translation: Although machine translation technologies are usually more easily available and simpler to operate, they usually lack sophisticated features and integration capacity. They work better for quick, one-time translations than for long-term projects that contain a lot of texts.
Conclusion
While both AI translation and machine translation play essential roles in the language services sector, their capabilities and applications vary greatly. AI translation is a more advanced option that provides translations that are adaptive, accurate, and aware of their context—perfect for professional translations. Even though machine translation is more basic, it can still be useful for simple translation tasks and quickly understanding material written in a foreign language. As technology advances, the gap between these two approaches may lessen, but recognising their distinctions is critical for selecting the best solution for certain translation requirements.